This month we ask “What are your thoughts on the future of artificial intelligence (AI) in diagnostics?”
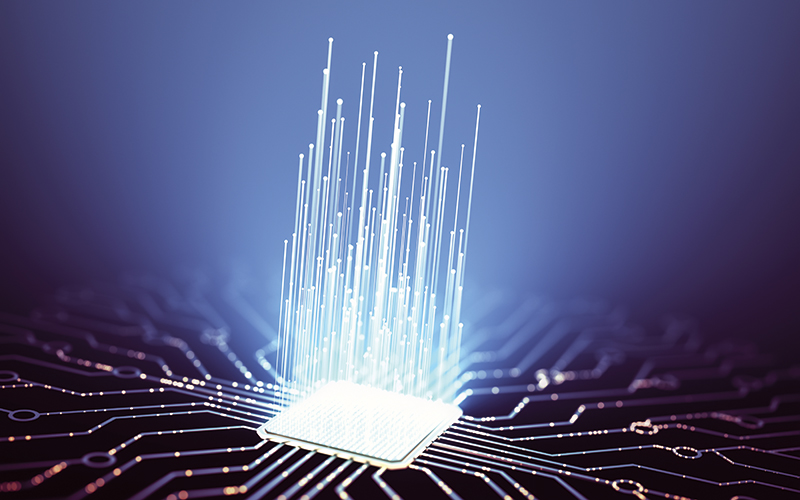
Paul Chenery
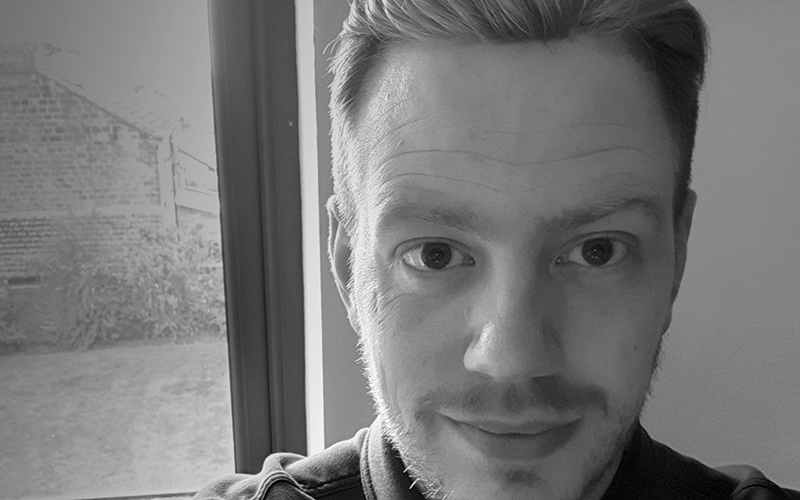
Quality, Training & Transformation Manager – Cellular Pathology
Nottingham University Hospitals
As a profession it is vital that we do more to deliver a sustainable service that is fast, affordable, and reliable, but most of all that meets the needs of all our patients.
The rise of primary digital reporting and whole-slide imaging for cellular pathology has opened the door on many possibilities, and should be seen as more than just a digital microscope, but rather a chance to transform service to patients.
AI algorithms, and their use, are just one of the many exciting development possibilities. Standardisation and reproducibility are the cornerstones of laboratory practice. AI is able to produce the same results again and again unaffected by external factors (emotion, distraction, level of exhaustion etc.). Machine learning provides a platform where the more it is trained, the more accurate it becomes. However, if a flaw exists within a programme, that same flaw will happen every time.
What does the future hold? With a national shortage of histopathologists we have to look for a different way of working. Perhaps with investment and willingness AI could be integrated to screen “normal” slides, allowing trained pathologists to concentrate on more complex cases.
This is a huge leap in current practice and would require significant regulation from professional and accreditation bodies. An open mind is needed with AI being used as a companion diagnostic tool rather than for workforce replacement.
Chloe Knowles
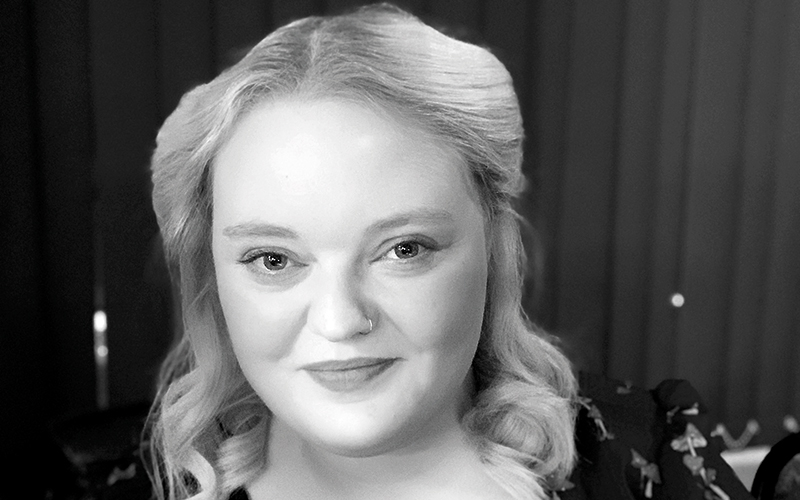
EMEA Laboratory Workflow Optimisation Consultant
Leica Biosystems
Artificial intelligence has an important role to play in diagnostics as we see increases in workload, complexity of cases, and the advancements of diagnostic testing. Due to current pathologist and laboratory staff shortage, there is additional pressure on the current workforce to ensure patients receive their result in an appropriate time frame whilst maintaining high quality.
The implementation of both digital pathology and AI into cellular pathology for diagnostics could support pathologists and biomedical scientists with triage, by identifying cancer vs. non cancer cases before they leave the laboratory. This can allow prioritisation of cases, giving pathologists more time to review complex cases and focus on patient outcomes.
AI could complement the complex diagnostic pathways with the introduction of suitable algorithms to score companion diagnostics, however, we need to ensure that AI is perceived as a tool to facilitate efficiency and quality in the laboratory, not as a replacement for laboratory staff or pathologists. To prepare for artificial intelligence and instill confidence in the results it produces, we need to enable successful digital pathology, which means reducing the pre-analytical variability that exists within each step of the histopathology workflow. High-quality slides produce high-quality images, leading to better digital pathology outcomes and confidence in artificial intelligence.
Dr Owen Driskell
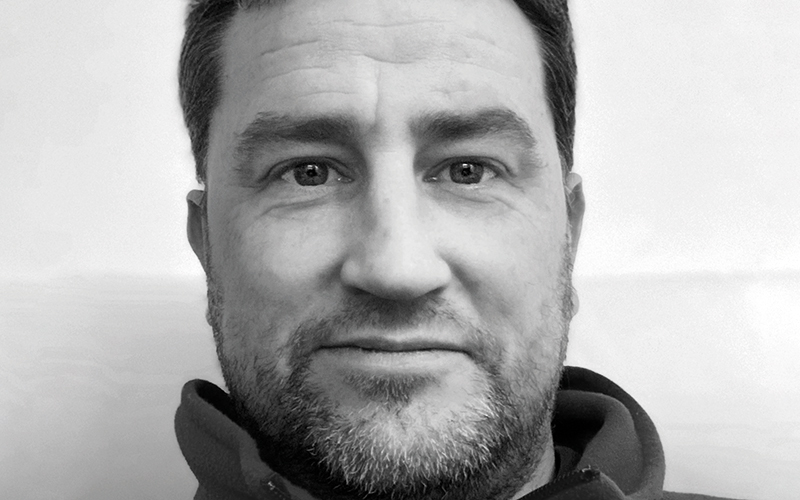
Deputy Training Programme Director for HSST
National School of Healthcare Science
Artificial intelligence technology aims to create applications capable of performing tasks commonly thought to need human intelligence. It is essentially using computing power, potentially enormous data input and some clever computer science to calculate probabilities and statistics, and recognise patterns. Patterns that humans might not even be able to discern. Indeed, it can be more successful when you don’t try to teach the application what you are looking for, but let the application learn for itself, so-called machine learning.
In diagnostics this approach has been used to develop applications for recognising from images when a biopsy shows signs of cancer. Data inputs vary depending on the question posed. Blood test results may be part of the data input for an application to recognise patients at risk of sepsis. These are just two examples where pathology outputs become inputs for AI and in a way become pre-analytical considerations themselves.
In diagnostics an understanding of the pre-analytic factors together with an understanding of the methodology used for the analysis supports the interpretation of the result. For AI it is no different. The careful curation of digital data input and a knowledge of how the AI operates supports its use as a tool in decision-making. It is these skills and knowledge that health professionals will need to master.
Image credit | iStock